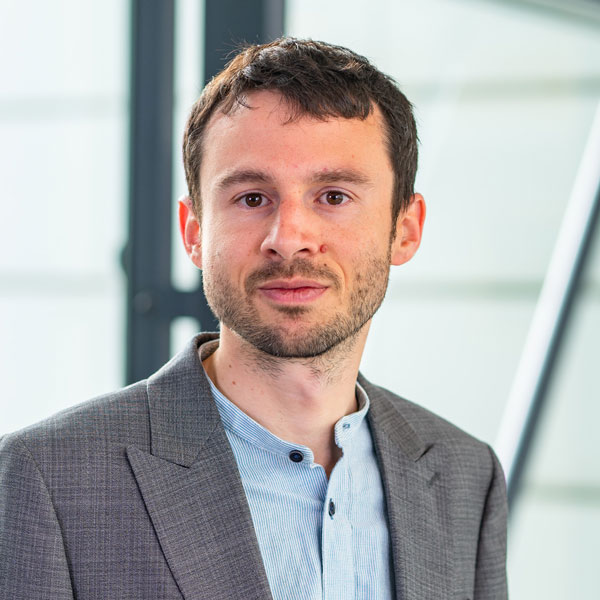
Emmanuel Hardy
Bio:
Emmanuel Hardy is a research engineer in the Integrated Circuits for Power Management, Sensors and Actuators Lab. He joined CEA Leti in 2020. His research interests cover ultra-low power circuit design for sensor signal processing and small footprint neural networks. He previously designed mixed-signal circuits and algorithms for audio applications at Wolfson Microelectronics and Cirrus Logic (Edinburgh, UK).
He holds a Master’s degree in Microelectronics from the Ecole des Mines de Saint-Etienne (2009) and a PhD in Electrical Engineering from Aix-Marseille University (2013).
Abstract:
Sensor arrays impose significant constraints on the power budget of battery-powered smart sensors due to redundant analog front-end, analog-to-digital conversion (ADC), and digital signal processing for each channel. However, by leveraging the spiking domain for converting and processing relevant information, energy consumption can be reduced by several orders of magnitude. This principle can be extended to various sensor data.
In this talk, we present the groundbreaking development of an end-to-end ultra-low power Gesture Recognition system. Our system comprises an array of emitting and receiving piezoelectric micromachined ultrasonic transducers (pMUT), along with driving/sensing electronics. To extract distance and angle information from incoming echoes without conventional ADCs, we propose a novel spike-based beamforming strategy. Additionally, we employ a Spiking Recurrent Neural Network for Gesture Recognition, achieving a classification accuracy of 86.0% on a dataset of five 3D gestures using our setup.
Furthermore, we demonstrate the effectiveness of our approach in object localization, inspired by the barn owl auditory system. Leveraging ultrasonic data from two pMUT sensors, our signal processing and detection mechanism consumes 3000 times less power compared to a lightweight algorithm implemented on a MCU.
By harnessing these innovative methods, we pave the way for the development of tomorrow's smart and low-power devices, revolutionizing the possibilities for energy-efficient sensor applications in various domains.
Thursday Memory for Edge Computing PM
Research Engineer, CEA-Leti
Ultra-low Power Edge AI: Bridging the Gap between Sensors and Neural Networks... more info